Is it possible to inventory a 1,000 km bike network in just over a week? With RoadAI, it is.
Montréal is a great place to bike, thanks to its excellent network of bike lanes that spans over 1,000 km (625 mi)—one of the largest and most bike-friendly in North America. The Express Bike Network (EBN), also known as the Réseau express vélo (REV), serves as the backbone of Montréal’s cycling infrastructure and is designed for year-round accessibility. Of the network, about 350 km consist of dedicated bike paths or bike paths protected by concrete barriers and poles, while about 700 km are marked bike lanes with ground markings and signage.
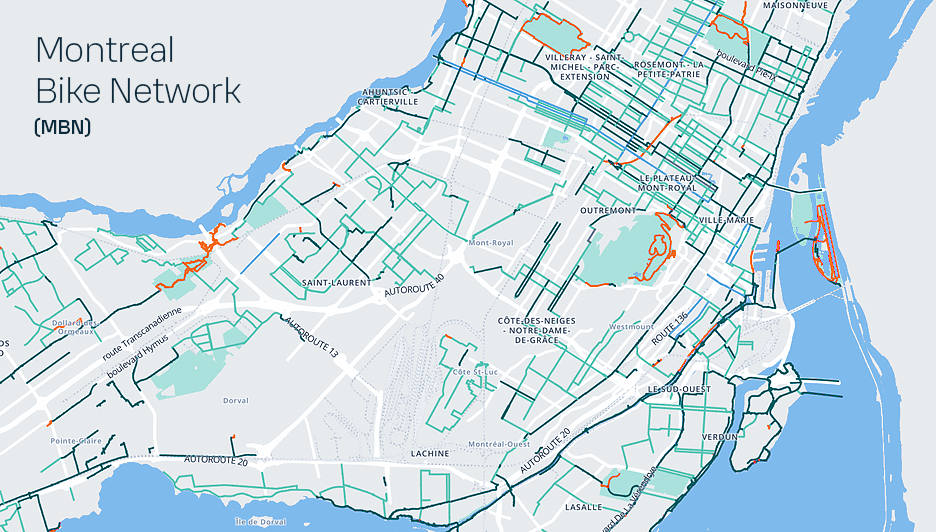
Knowing the condition of this network is an important part of the process in managing it, determining needs and establishing an adequate level of service for the city's users. However, this extensive network includes several types of lane, from cycle lanes to dedicated tracks, as well as pavements and shared lanes, which poses problems of accessibility for inspection vehicles.
The automated cycle network inspection project launched by the City of Montreal therefore aims to:
- Improve accessibility: using an active means of transport to monitor the entire cycle network
- Establish a unified methodology: adopting an automated, high-performance method
- Enable cost savings: the newly deployed equipment and technologies are expected to save an estimated two to three times their cost.
In order to unify surveying methods, the City deployed RoadAI, an AI-powered automated road asset inventory tool that has been highly successful for road network management organizations globally. Although the tool is originally designed to survey driveways, the City of Montréal successfully adapted the solution (which can be used on a regular smartphone) to survey the bike paths across the network in one go. This was made possible thanks to Ariane Garon, a Master’s student in Geography and Geomatics at the University of Montréal. For over a week, Ariane became "the eyes of the city," collecting road condition data across the 1 600 km of bike lanes on her electric bike using RoadAI.
Video by Radio Canada
Originally published by CBC News, Elias Abboud, June 27, 2024
Ariane is also assisting with the post-processing of the collected data, under the supervision of Nam Nguyen, an engineer in the City of Montreal's Strategic Management Division.
Below, Nam Nguyen, Eng., Ph.D., Engineer, Department of Strategic Asset Management, the City of Montreal, shares his experience deploying and using RoadAI.
Smooth integration & comprehensive support
According to Nam, the solution exceeded his expectations. From deployment to the compilation of results, RoadAI seamlessly met the city's needs and integrated smoothly into existing methodologies. Comprehensive technical support throughout each stage of the project ensured a smooth and efficient deployment.
"While surveying the network, I was pleasantly surprised by the ease of use of the app on an Android phone. Data management and transfer, once collected in the field, happen automatically in the background, saving significant time and reducing the potential for errors," says Nguyen.
Powerful features
In processing mode, Nam particularly appreciates the tool's rich functionality. For instance, users can create a customized interactive map that incorporates several features: survey data management, thematic maps in both survey mode and compiled mode, all tailored to the client's repository.
“We are pleasantly surprised by how easy it is to use the RoadAI app. In processing mode, we appreciate the convergent results obtained with AI and the enriching, well-engineered features.”
Nam Nguyen, Eng., Ph.D., Engineer, Department of Strategic Asset Management, the City of Montreal
As a cloud-based solution, RoadAI leverages AI to automatically process and identify pavement defects, delivering results consistently within 48 hours. Nam expresses his appreciation for the development team, highlighting their ability to integrate robust GIS methodologies and the successful integration of the city’s repository into the tool.
Effective reporting functionality
In report mode, the City of Montreal utilizes two types of reports: a detailed report with a resolution up to 5 meters, and a section assessment, both of which align perfectly with the city’s needs. Furthermore, users can continuously track not only the quantity of identified deficts but also their precise locations in each analyzed image. "We find that the modules for detecting potholes, road markings, and street furniture offer highly valuable insights," adds Nam.
Next in the project, the city will validate the results obtained from RoadAI compared to the manual and semi-automatic methods; after that, performance indicators for cycle lanes with be developed. All the results obtained will be published on the Montreal Open Data Portal.